Image Anything: Towards Reasoning-coherent and Training-free Multi-modal Image Generation
-
Yuanhuiyi Lyu
AI Thrust, HKUST(GZ)
    -
Xu Zheng
AI Thrust, HKUST(GZ)
    -
Addison Lin Wang
AI Thrust, HKUST(GZ)
Dept. of CSE, HKUST
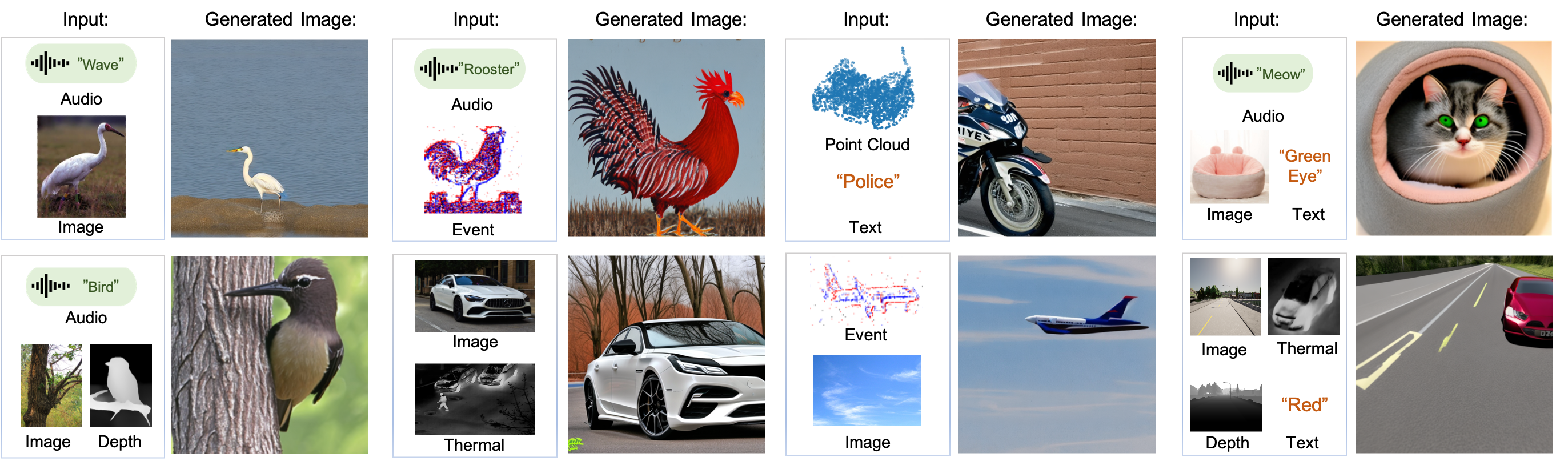
Abstract
The multifaceted nature of human perception and comprehension indicates that, when we think, our body can naturally take any combination of senses, a.k.a., modalities and form a beautiful picture in our brain. For example, when we see a cattery and simultaneously perceive the cat's purring sound, our brain can construct a picture of a cat in the cattery. Intuitively, generative AI models should hold the versatility of humans and be capable of generating images from any combination of modalities efficiently and collaboratively. This paper presents ImgAny, a novel end-to-end multi-modal generative model that can mimic human reasoning and generate high-quality images. Our method serves as the first attempt in its capacity of efficiently and flexibly taking any combination of seven modalities, ranging from language, audio to vision modalities, including image, point cloud, thermal, depth, and event data. Our key idea is inspired by human-level cognitive processes and involves the integration and harmonization of multiple input modalities at both the entity and attribute levels without specific tuning across modalities. Accordingly, our method brings two novel training-free technical branches: 1) Entity Fusion Branch ensures the coherence between inputs and outputs. It extracts entity features from the multi-modal representations powered by our specially constructed entity knowledge graph; 2) Attribute Fusion Branch adeptly preserves and processes the attributes. It efficiently amalgamates distinct attributes from diverse input modalities via our proposed attribute knowledge graph. Lastly, the entity and attribute features are adaptively fused as the conditional inputs to the pre-trained Stable Diffusion model for image generation. Extensive experiments under diverse modality combinations demonstrate its exceptional capability for visual content creation.
Overall framework of our ImageAnything
The overall framework of ImgAny, which includes: 1) Multi-modal Encoder, 2) Entity Fusion Branch, and 3) Attribute Fusion Branch.
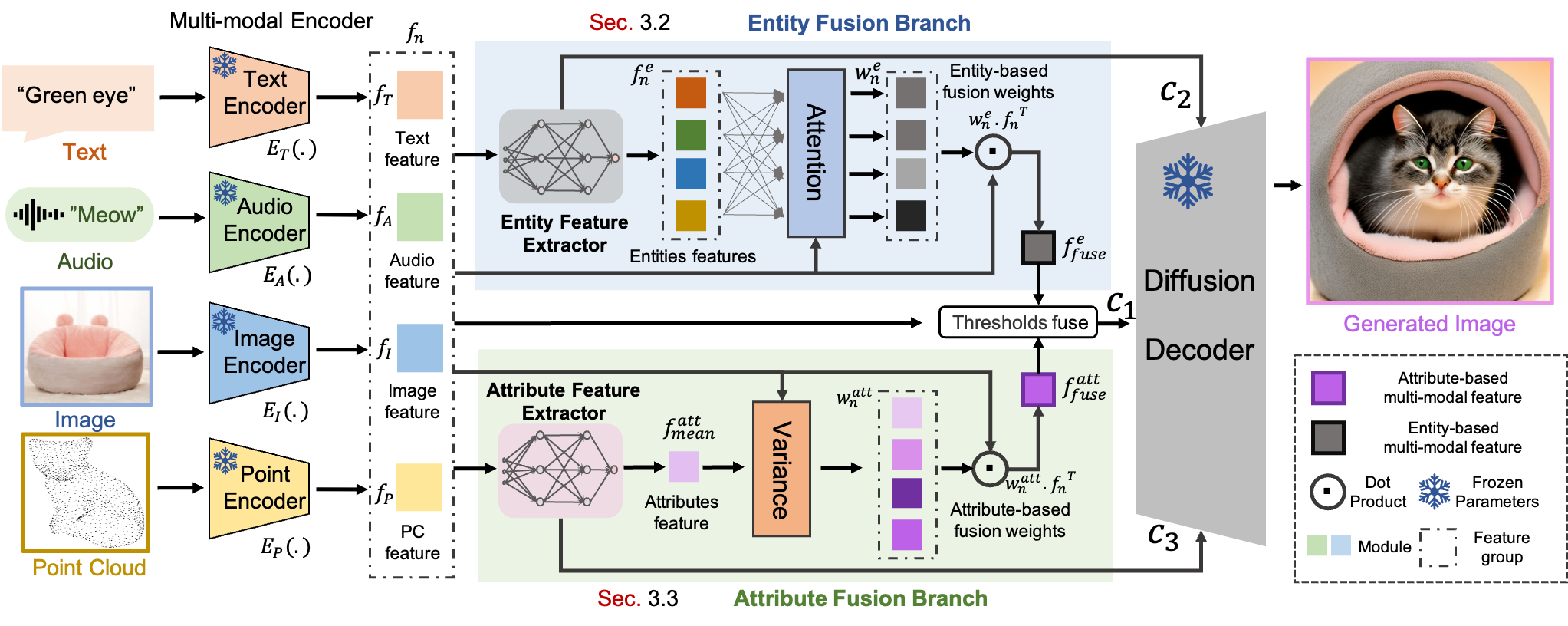
Qualitative Comparison with CoDi and Baseline
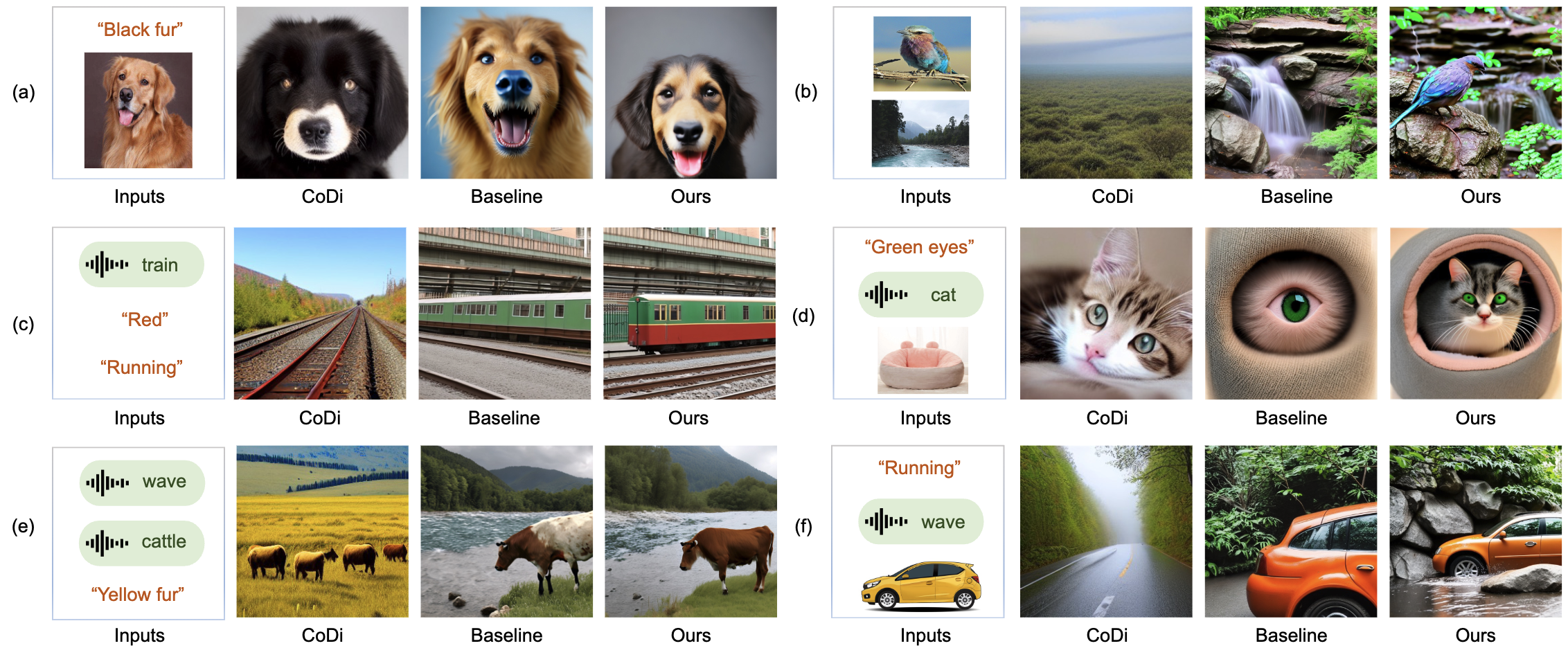
Qualitative Comparison Focuses on Seven Modalities
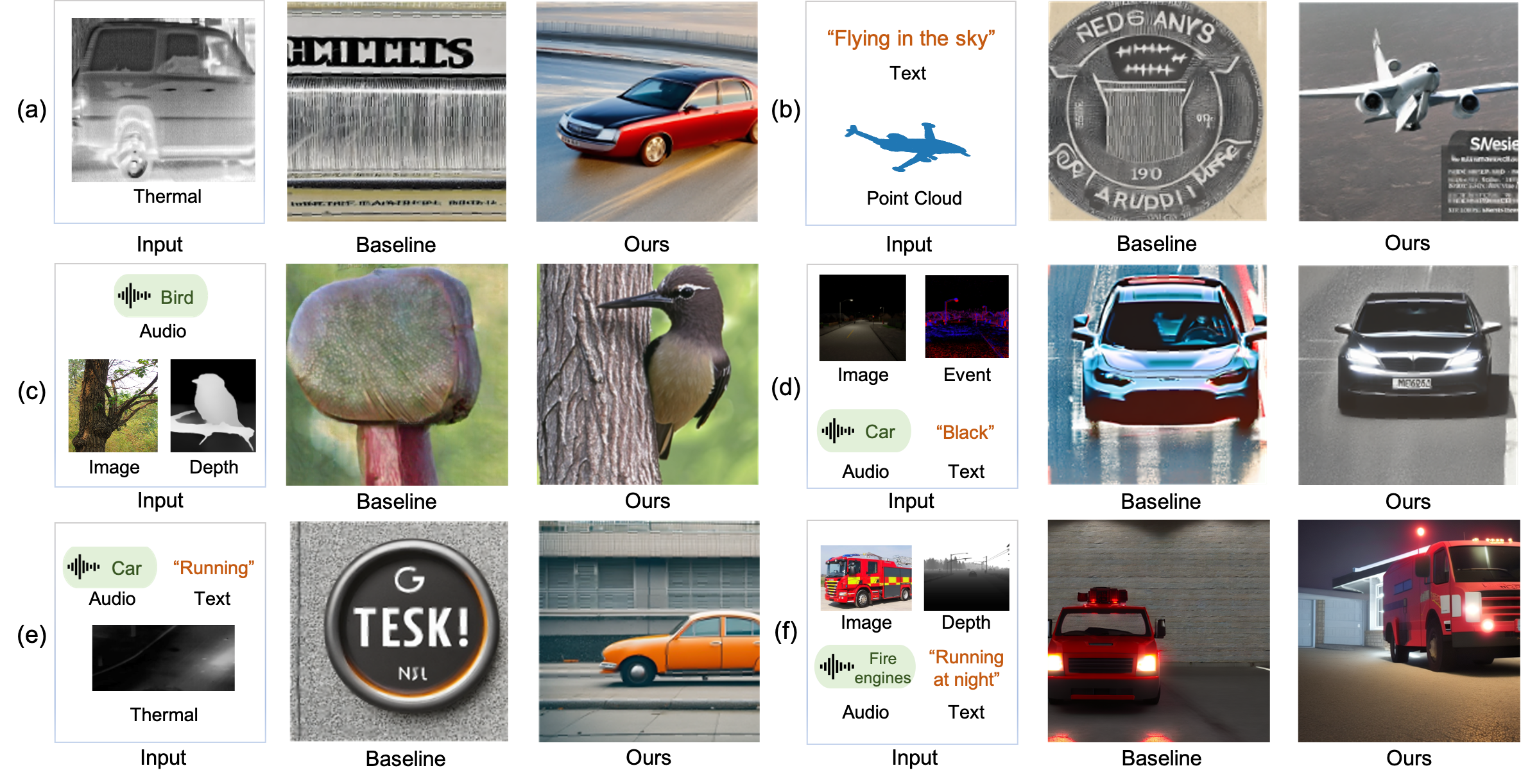
Additional Results (Any combination of text, audio, and image modalities)
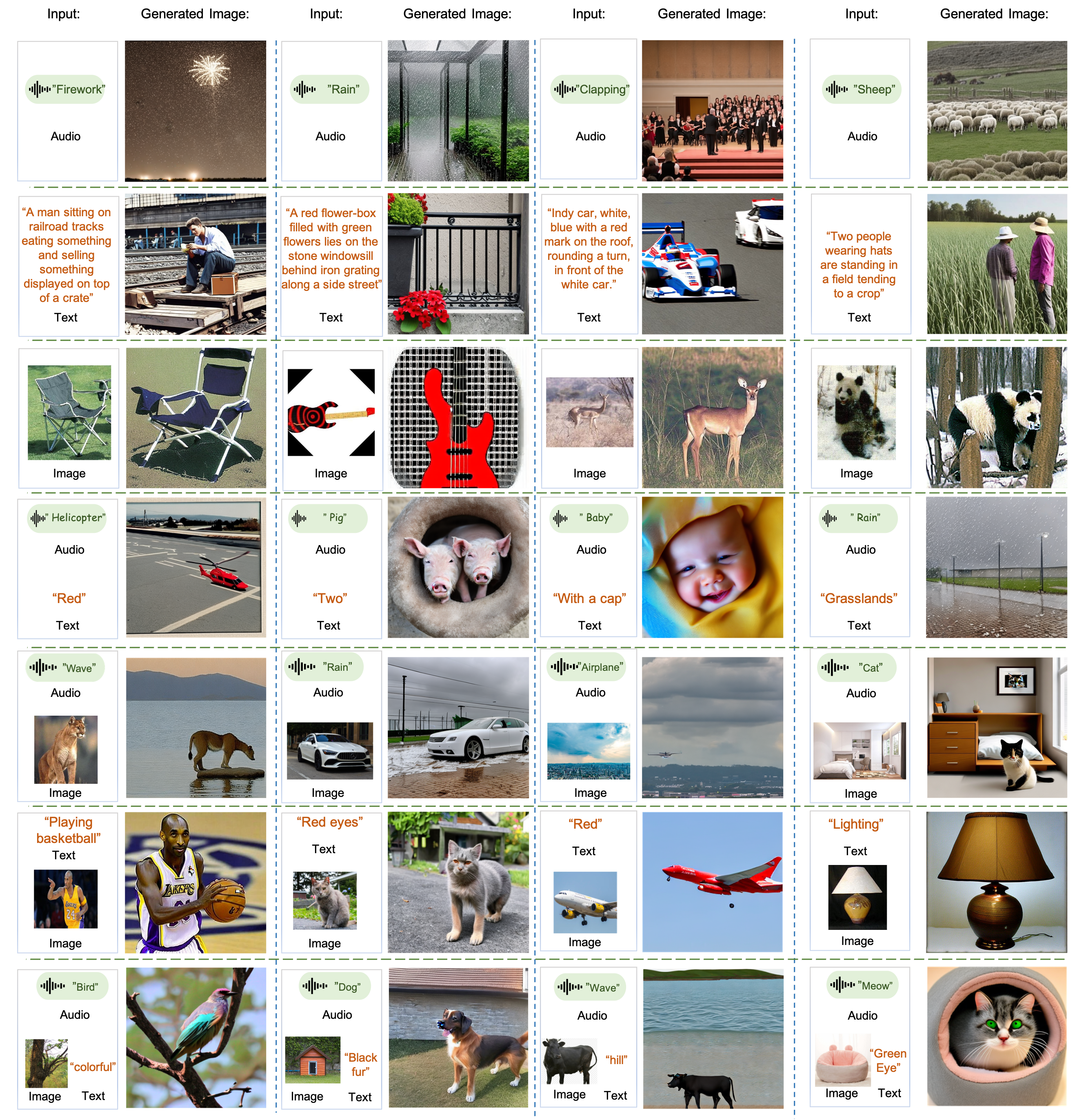
Additional Results (Any combination of seven modalities)
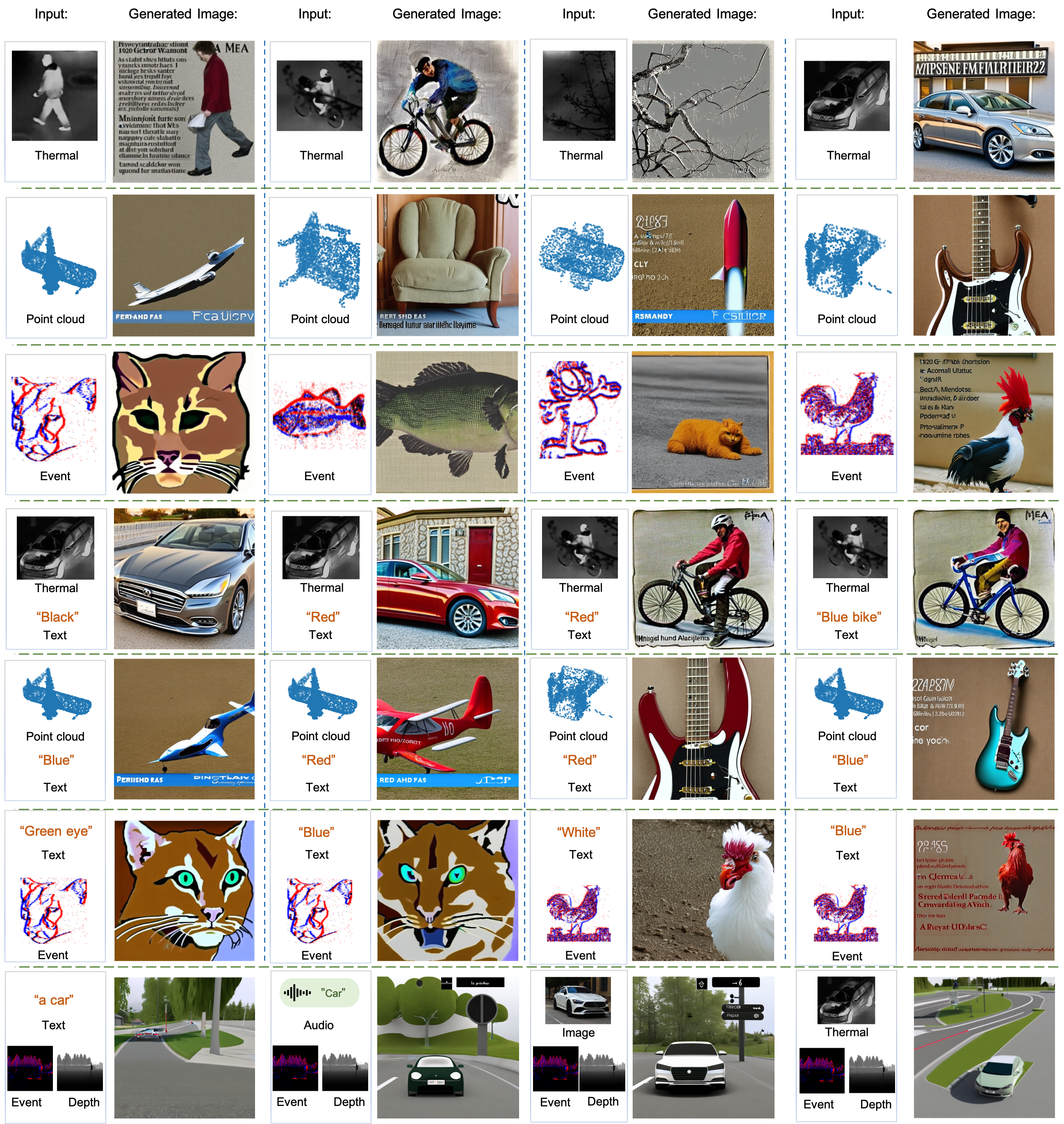
BibTeX
@article{, title={Image Anything: Towards Reasoning-coherent and Training-free Multi-modal Image Generation}, author={Yuanhuiyi Lyu and Xu Zheng and Lin Wang}, journal={arXiv}, year={2024} }